Brought to you by Kate Strachnyi | DATAcated & Ravit Jain | The Ravit Show
Jam packed full of jam (will explain later)
2 day event | 14 speakers | 10 minutes each (+ Q&A) | Surprise Guests!!
These sessions went by lightning fast, so if you missed out on any second of this rad event, do not fear, check out my summary below.
As with any great story, it is critical to start with an origin story. And with any great origin story comes a detailed explanation, typically viewed as pure awesomeness. Just a couple of months prior to this day, Kate and Ravit joined forces to put on a full data party dedicated to solving modern data problems. Ravit came up with an awesome sweater concept (worn by Kate) and after gathering a wonderful array of sponsors and community partners, the Modern DataFest was a go!
Day 1
Behavioral Data Creation for AI - Delivering Value & Insights
The new bees knees has been around this concept of creating data. Rather than trying to turn existing data into something more useful which hopefully leads to insights and is successful in providing business value, why not just create the data. Nick talks about how data creation is central to unlocking competitive advantage as it has the ability to create AI and data applications at scale.
Specifically, data creation is the process of deliberately creating data in your data warehouse or lakehouse, and is AI and BI-ready by design. How? It is reliable, explainable, accurate, predictive, and compliant. Magnificent!
Many of you know the 80/20 rule with data, where 80% of the time is dedicated to data preparation and only 20% of time is left for the good stuff, creating and sharing insights which lead to business value. When you swap your data collection model to data creation, you also swap this rule. Now only 20% is required for the data creation (this includes the old school prepping) and a large 80% of your time is now dedicated to creating insights and providing business value through data applications. Yay!!
The other super cool part about data creation is that it can be used across many different industries. Check out some of the neat things you can do:
Media & Entertainment: predicting user churn and intervene with subscription offers
Retail: Real-time customer personalization
Technology: Create rich customer 360 profiles
Financial Services: Train AI and ML models to detect and prevent fraudulent activity
Healthcare: Protect sensitive data with secure deployment
I don't know about you, but I am fully on board with this data creation business.
Who's with me 🙋🏻♀️
The Sixth Force - Data Driven Competitive Advantage
Before getting into Vinay's invention of the sixth force, we have to backtrack a bit and review Porter's 5 Forces for the newbees in the back (including myself).
Porter's Five Forces is a model that identifies and analyzes five competitive forces that shape every industry and helps determine an industry's weaknesses and strengths. They are as follows:
Competition in the industry
Potential of new entrants into the industry
Power of suppliers
Power of customers
Threat of substitute products
As time progresses, competition have become more fierce. Thus, the need for a sixth force: A Strategic Data Strategy
Vinay shared a statistic by Gartner, which states that only 20% of analytic insights will deliver business value. That means that 80% of data projects fail!! Looks like the 80/20 rule can be used in many contexts... ah yes, The Pareto Principle
Vilfredo Federico Damaso Pareto was born in Italy in 1848. He would go on to become an important philosopher and economist. Legend has it that one day he noticed that 20% of the pea plants in his garden generated 80% of the healthy pea pods. This observation caused him to think about uneven distribution. He thought about wealth and discovered that 80% of the land in Italy was owned by just 20% of the population. He investigated different industries and found that 80% of production typically came from just 20% of the companies. The generalization became:
80% of results will come from just 20% of the action.
But why do so many data projects fail?
Vinay shared some inhibitors to creating a competitive advantage:
No data strategy
Technology focus
Lack of tacet knowledge and IP capture
Business intermediated by data scientists & IT
Slow data integration
Lack of data trust - quality and governance
Single view only after centralization
While the data strategy is the first on the list and tied to the sixth principle, it was noted that data quality is an absolute staple and strongly advised to never use data if it lacks data quality. Great advice!
Vinay mentioned that he offers a half-day workshop so if you would like to learn more, connect with him on LinkedIn.
What you Need to Know to Pivot to MLOps
Mikiko's session was JAM PACKED with all kinds of MLOps goodies! (this is where the jam came from)
The presentation was mainly focused on sharing the 5 rules to MLOps, or as Mikiko phrased it, 'My MLOps Manifesto'
The stack that's right for you might not be right for others. And vice versa.
Boring stacks are where it's at but new tools might mitigate old problems.
The stack should address two outcomes: (i) serving innovative, value-producing models consistently and at scale (ii) employee retention. It is does netiher it's useless. If it does one it's incomplete.
The hardest problem to solve in MLOps adoption isn't tools. It's ego.
The 'right' MLOps candidates look less like Formula 1 Cars... & a lot more like Range Rovers.
The best part of this session was the candid view of how MLOps actually operates in the real world. A few epic call-outs:
We need to hire people, not titles
People can help themselves and adoption of good MLOps if they didn't have their head up their butt
Company FOMO: we need to make it more complex, we need more boxes!
But what about the JAM??
Jam was referenced to when talking about ALL the tools that make up the ecosystem these days. If you want to start with MLOps, 'where do I start' is the wrong question. You need to focus on the particular question you are trying to solve for first, and only then are you able to select the right Jam. I mean, check out this selection!!
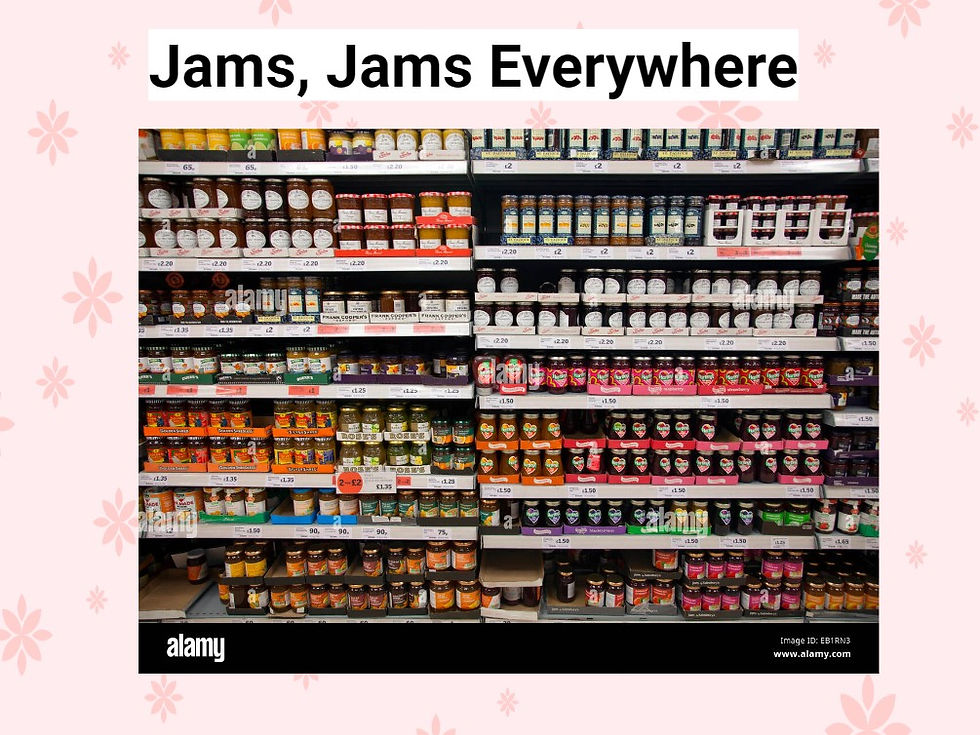
Mikiko's session was so popular that everyone requested the slides, and Mikiko delivered! Go check them out, there are a lot of fun memes!! 🤓
You can find them on LinkedIn and also on Google Docs
Modern Data Stack 2.0 vs Post Modern Data Stack. Same or Different?
This was a fun discussion around the naming of the Modern Data Stack.
Chris took us through the evolution of the data ecosystem, from the beginning (Data Warehouse - era) to the open source bubble (Big Data Platform -era) to the current rise of the cloud SaaS (termed: Modern Data Stack).
We have evolved so much in this space because there are newer and cooler technologies, mostly due to the growing complexity of current business problems.
The problem with the Data Warehouse was that is was mostly on premise. Therefore it was not available to everyone and required $$$ to scale.
The problem with the Big Data Platform was that it required an army of engineers with very high technical skills.
But what about the Modern Data Stack... the 'problem' is that we have been in this era for quite some time. So do we need to change the name?
The overall conclusion was, no. Not that things have remained the same all this time because we have been modifying and evolving, but for simplicity. We have just ran out of good names. Really... the proposed names were... not great
Modern Data Stack 2.0
Post Modern Data Stack
Modern Data Stack 10.3
Platform
Do you think the new modern data stack needs a new name?
If you are interested in learning more, check out Chris' LinkedIn post here
Data Modernization at High Speed
Saket and Sid were the last formal session, structured as a fireside chat. Sid shared his views of data modernization through his time at Bed Bath & Beyond. They adhere to the 3v's Framework (variety, velocity, volume).
In retail, there is a variety of data sets:
Customer data
Transactional data
Product data
Financial data
and a bit newer... Media data (how are customers engaging with the business)
In retail, the velocity of data is key. Customers are curious about the points they are earning on their purchases, which requires real time transactional data.
In retail, the volume of data is immense for both Ecommerce and Digital Experience as it can involve terabytes of data!
The end of this session was a quick and fun lighting round:
'Da-tuh' or 'Day-tuh' >>> 'Day-tuh'
Data Integration or Data in Place >>> Data Integration
Data Pipeline or Data Flow >>> Data Flow
Data Fabric or Data Mesh >>> Data Fabric
Unannounced Surprise Guest
This was a lovely treat, Tiankai Feng shared with us his massive creative talent by merging data, music, and fun as a 3 minute compilation of data themed musicals.
Here is a teaser:
Rent
'525,600 errors, 525,000 lines to review'
Hamilton
'Master Data Management, we work in Master Data Management'
Wicked
'It's time to try defining quality, before we fix, define what's quality'
Les Miserables
'On my own, with no real business context'
Little Shop of Horrors
'Suddenly Lakehouse, it's standing beside you'
Evita
'Don't cry for now it's just excel, not everybody learned to use SQL'
Cats
'Memory, we're running out of memory!'
West Side Story
'Somewhere, somehow, I lost the documentation'
The Wizard of Oz
'Somewhere there is a dashboard to be found'
Les Miserables
'Do you know what people need? Basic data literacy!'
For more, follow him on YouTube
Official Surprise Guest
Trivia + Giveaways
The official surprise guest was... Scott Taylor - The Data Whisperer!
Scott guided us through a bumpy game of Trivia, including questions from throughout the event, both Day 1 and Day 2. If you know Scott, he also provided loads of laughs. Like seriously, I could not even write down all his jokes this time to share with y'all. This was the exact moment that made the crowd bust out in laughter, just look at Kate and Ravit 🤣
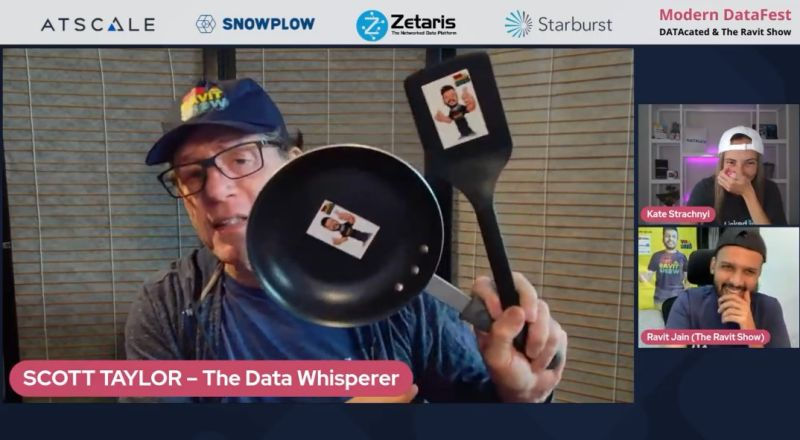
I did manage to grab a couple jokes:
meta data = data about data
meta meta data = data about data about data
Seinfeld data = data about nothing 😂
What chemical regulates a rabbit's mood 🐇
... carrot-tonin 🥕
To close out the day, Scott mentioned Kate's fun October video series, 'The Data is NOT Scary'. He participated in this series by talking about Master Data Management #MDM and included in his video there are a few scary movie clips. If you can find all of them, Scott will send you a copy of his book (DM him the movie clips to enter).
Check out the video here.
Way to go Kate and Ravi, Day 1 was a huge success. Now it's time for a nap 😴
I will be back again tomorrow to add Day 2.
Back! - Day 2
Rested, relaxed, and ready for another exciting day!
Some general observations from today's session:
> I really enjoyed the various wardrobe changes and now I'm wondering if this happened during Day 1 as well, haha!
Ravit's wardrobe included an AtScale t-shirt, representing their book: Make Insights Actionable with AI & BI and a t-shirt from trino, which showed an adorable bunny 🐰
Kate wore two different DATAcated shirts plus a sweatshirt loaded with Sponsors. She also showed off some pretty sweet socks from Dremio.
> There was a funny ongoing joke from yesterday, 'it is not officially a party until someone is on mute' 🔇
> Lastly, I think there is a real need to create the Ravit cookware that Scott presented yesterday. Scroll back up if you are curious, I included a picture that speaks 1,000 words 🤣
💻 Now back to the regularly scheduled programming
Gaurav Rao - How to Leverage a Semantic Layer for Applied AI
The main point of this session was to share two numbers: 500 and 54
The International Data Corporation (IDC) predicts that in 2023, the annual AI investment and spend will hit $500 Billion
One Gartner study related to the production use of AI found that only 54% of AI models move into production!
If we are spending so much on these efforts, why do we keep failing? Gaurav mentions that it is because AI is still very complex and lacks business adoption. If predictions and insights are not being applied to business use cases or business problems, that ML model and/or AI project is never going to see the light of day.
The good news is that we still have the opportunity to help with the adoption, but there will be challenges. 3 words: relevant, consumable, explainable
In order for a business user to use ML or AI predictions, they need to be relevant. ML and AI currently exist in separate environments; therefore, we need to make these predictions consumable for business users. One solution could be with BI dashboards. Lastly, the business is not going to understand anything if the solution is not explainable, in business terms.
Now comes the sales pitch... the solution is the Semantic Layer! Check out my review of the Semantic Layer Summit if you would like to learn more.
Tom Nats - Why Rent When You Can Own? Build Your Modern, Open Data Lakehouse
If you are still new to the lakehouse concept, a lakehouse is basically a data lake on steroids. The idea of a data lake a decade ago was to throw everything into the lake, which looks like a garbage heap. But this is no longer the case as the modern day lakehouse looks more like an organized closet, providing structure and security.
Some misconceptions about lakehouses:
Lakehouses are like data lakes with bad data, not for BI reporting, and require special skills. In reality, modern lakehouses use table formats which force structure and when used with the right tools can interact with the data using your favorite existing tools.
And most importantly, the data is in your account under your control, in your account!
Why is this a big deal? Because it's your data. It should be free to be used by anyone without being held hostage. This is why instead of referring to data as 'oil', Tom likes to look at it as electricity.
And to end with a SCARY quote:
Innovate or we're dead
Dr. Serena Huang - Innovation in People Analytics
I have said this before, but I will say it again. People Analytics is one of my favorite topics, it is completely fascinating with its endless possibilities. If you want to learn more about these possibilities, Serena teaches a course: The Data Science of Using People Analytics, available on LinkedIn Learning.
At a high level, people analytics are used to improve decisions about people. It is not only for HR, it is for the entire company. As with the marketing customer conversion funnel, people analytics use something similar while converting candidates to new hires.
Serena shares her 3 pronged approach to innovate people analytics:
Learning-Focused
Customer-Obsessed
Modern Tech-Powered
Learning is key to being successful in the space of innovation. Whether that be learning something new, relearning something that you forgot, or unlearning something that no longer applies.
The illiterate of the 21st century will not be those who cannot read and write, but those who cannot learn, unlearn, and relearn ~ Alvin Toffler
Customer-obsessed sounds a bit strong IMO, but the focus here is on relationship building. This is also key to being successful in the space of innovation because that is how you build trust from your customers.
Lastly, you cannot begin to consider yourself in an innovative state if you are not thinking about using modern technologies.
Dipankar Mazumdar - Apache Iceberg: An Architectural Look Under the Covers
I like the title of this talk, 'look under the covers'. Icebergs are commonly referred to when talking about data products. The tip of the iceberg represents the final product as that is what everyone sees. However, if you 'look under the covers', you find the bulk of the iceberg, where all the behind the scenes effort, struggle, and magic happens.
Dipankar starts with the evolution of data architecture going from Data Warehouse to Data Lake to Data Lakehouse. He describes the Data Lakehouse as a merge of the previous architectures, using the best parts of each. As it is beneficial to have all data dumped in one location, it needs to have structure to be able to use the data.
The Apache Iceberg is the key technology for lakehouse as it is designed to deal with large-scale data and effective query planning. It also offers many capabilities, including table format specification and a set of APIs and libraries for interaction with that specification.
If you would like to learn more, check out the official iceberg page
Vidhi Chugh - Key Elements of a Winning AI Strategy
Vidhi discusses two key components:
The need of a Strategy
Focusing on an AI Strategy
A strategy is basically a roadmap to your strategic goals. This includes completing projects that will help you to attain those goals. But not just any projects, there are a ton of projects that you can be doing. It is essential that you pick the right project(s) to work on and if there are more than one, prioritize appropriately.
With typical projects, the focus is on the implementation rather than the design. When AI comes into the picture, this is still mostly the case but you need to understand that the primary focus is on the data. Note, the availability of the data is not the important part as you need to make sure that you are using the right data, it is of good quality, and it exhibits some type of pattern (this is what fuels AI models).
To be successful in winning, it is critical to ask some important questions up front. Who are the end users, that impacts would an incorrect solution have on those users, does the project cut across several verticals or can it be done by one particular team. And maybe most importantly, is the project even scalable.
The last takeaway I had with this discussion was the importance of evangelism. The more you see the adopters hop on board, you will see an increase in confidence from the project team that is working on the solution.
Li Kang - Building a Modern Analytics Platform
There has been a shift of analytics' roles in today's modern world. What used to be heavy strategic goals is now expanding into strategic AND operational realms. This includes the shift of pure batch based data loads to including real-time (or near-real-time) data. We used to provide solutions mainly for internal users, but today we are able to offer more to external users as well. Lastly, what used to be standalone products are now embedded into things like websites and daily workflows.
The coolest part about this shift are the capabilities of creating real-time (or near-real-time) analytics. We have seen these analytics becoming more popular with customer and transactional data, like when someone wants to know how many rewards points they have to use or in detecting fraudulent activity.
We asked a nearly impossible question to Li at the end as we were curious about the % of companies that actually have a modern analytics platform. As expected, the statistics are unknown but Li predicts this to be low.
Salma Bakouk - The Future of Data Reliability
Salma comes from a company called Sifflet, which is the French word for 'whistle'. She gets this question so often that she is considering adding it to the Q&A page on the company website 😂
The real topic for today was Full Data Stack Observability. Data observability is a pretty new term, which is a collection of signals and metrics that can help data producers and data consumers get an idea of the health status of data assets.
It starts with the traditional data engineering folks. They are responsible for most of the data collection, loading, transforming, etc. But like many other roles, data engineering is a constantly evolving field and the modern data stack has helped automate a lot of data engineering workflows. However, there are some problems with this approach as it was found to be manual, not scalable, and time consuming resulting in increased silos and lack of ownerships. Additionally, the automation of the alerts created too much noise (including false positives) and alert fatigue that could be harmful to the organization if a true alert is missed.
This is where the term DATAtastrophies was brought up, we need to avoid these at all costs!!
The future of this Full Data Stack Observability concept is predicted to be powerful by providing:
Actionable Monitoring: knowing when and where the data breaks
Root Cause Analysis & Incident Management: identify the source of the issue and quickly asses the business impact
Cost Optimization: optimize infrastructure and resources
Mico Yuk - The Art of Data Collaboration: How to Reduce Analyst Workflow by 75%
The last session of the Modern DataFest was a FANTASTIC FINALY!!
We all have had experiences with collaboration. Typically, it is done through several meetings, gathering business requirements, completing other cringy tasks, and ending up with some kind of end product which is usually a dashboard of some sort.
There are several articles out there that claim dashboards are dead. That is not really the case, people still request them, A LOT. Something that Gartner clarifies is that dashboards are actually a dead-end and you have probably seen this happen. You spend all this time and labor creating a lovely dashboard with all the bells and whistles requested by your customer only to have it never be looked at again.
Mico does not think dashboards are dead, she does believe that they are useful. She also shares that the most useful dashboards should:
Tell stories
Include embedded content
Have a set narrative
Include annotations
Solve the real business problems
If you are 'lucky' people will have questions. I say 'lucky' because this usually does not end well. You can quickly find yourself drowning in the vast amount of questions upon questions, upon repeat questions because you have a thread that no one is reading, only responding. This is because no one knows how to collaborate!
Collaboration tips:
#1: BE HUMAN!
Often times you will find, especially in the corporate world, a bunch of robots. Once you have an end goal in mind, it's like that is the only focus without understanding or acknowledging the environment.
Collaboration should not be forced
You can to get into congruency as soon as possible, which increases the likelihood of other people agreeing with you. Mico shared what she calls The 3 Bods. Basically, you want to start a conversation with your collaboration group by asking a single binary question (yes or no). Hopefully their answer is Yes. You only need two more and you achieve congruency!
Be less focused on technology and more focused on the person
This is related to being human. Technology itself is not going to solve all our problems.
The most common problem that harms collaboration is, again, this concept of being human. People need to go into meetings with the right mindset, not only knowing the scope and purpose but also when to realize the meeting is no longer productive and it needs to be stopped.
Book Recommendation: Let's Get Real or Let's Not Play by Manhan Khalsa and Randy Illig
2023 LFG!
In Conclusion: I won!!
What a way to end the day and to end an enlightening event!!
The very last giveaway of the day, #THERAVITSHOW
Can't wait for my swag
Thank you!! 🤓
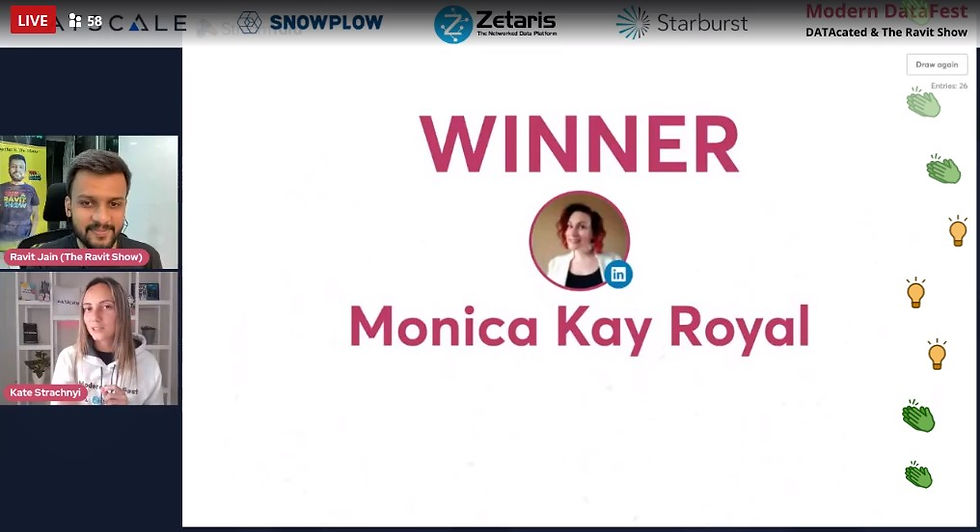
Happy Learning!!
Sponsors:
Community Partners:
Comments